In What Ways Does Machine Learning Contribute To Personalisation In Web Development?
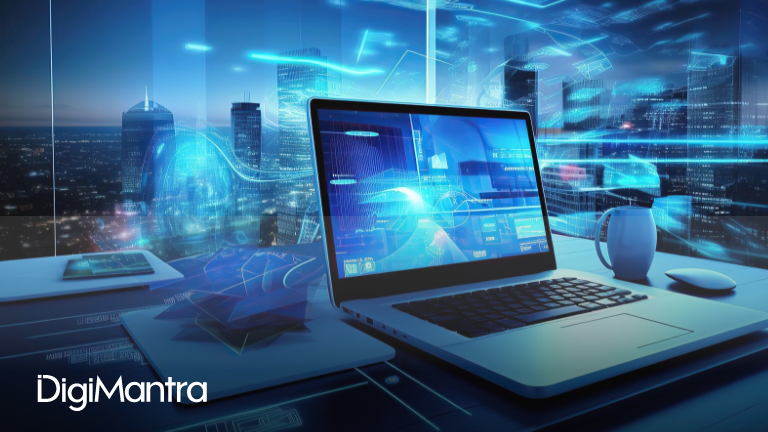
Introduction
73.1% of designers attribute visitor departure to non-responsive design. Machine learning is pivotal in enhancing personalisation within web development, revolutionising user experiences across various platforms. The machine learning market, valued at USD 38.11 billion in 2022, is forecasted to exceed USD 771.38 billion by 2032, with a projected CAGR of 35.09% from 2023 to 2032. One prominent contribution is through recommendation systems, where algorithms analyse user behaviour to suggest personalised content, products, or services. These systems leverage data such as browsing history, preferences, and interactions, tailoring suggestions to individual users.
Additionally, machine learning enables dynamic content optimisation, adapting website layouts and features based on user preferences. It ensures a more personalised and engaging interface, enhancing user satisfaction. Personalised search experiences are another aspect where machine learning algorithms refine search results based on user behaviour, leading to more accurate and relevant outcomes. Moreover, predictive analytics powered by machine learning anticipates user needs, enabling websites to present relevant information proactively. This proactive approach contributes to a more intuitive and user-centric web experience, fostering user loyalty and increased engagement.
Understanding Personalisation in Web Development
Delving into web development, personalisation transcends the simplistic act of addressing users by name; it encapsulates creating an online experience harmoniously attuned to individual preferences and behaviours. Unlike traditional methodologies governed by static rules, the advent of machine learning heralds a paradigm shift towards a dynamic and data-driven approach. At its core, machine learning (ML) leverages sophisticated algorithms to scrutinise extensive datasets, encompassing user interactions, preferences, and historical data. ML discerns intricate patterns through this analytical lens and formulates predictive models, empowering websites to comprehend user preferences and anticipate and respond to their needs
In the evolving web development landscape, machine learning is a catalyst for fostering a genuine sense of individuality in the online space. The intricate analysis of diverse data sets enables ML algorithms to unravel nuanced user behaviours, enabling websites to dynamically adapt and tailor their content and features. This departure from static approaches signifies a progressive move towards an adaptive and personalised digital environment. Ultimately, machine learning’s capacity to glean insights from vast data reservoirs contributes significantly to transforming user experiences, shaping the internet into a realm where individual preferences are acknowledged and seamlessly integrated into the fabric of the online journey.
User Profiling with Machine Learning
At the core of personalised web development, user profiling emerges as a pivotal component, and machine learning is its adept orchestrator. Demonstrating prowess in constructing intricate and dynamic profiles, machine learning algorithms delve into user behaviour analysis. It transcends the surface of basic demographics, as these algorithms meticulously consider browsing history, click patterns, and time spent on diverse pages.
What distinguishes machine learning in user profiling is its commitment to continuous learning. Through an iterative process, these algorithms refine and adapt user profiles over time. This adaptability ensures that recommendations and personalisation efforts align with users’ evolving preferences and behaviours. Machine learning empowers web developers to offer a meticulously tailored experience by maintaining a keen awareness of user interactions. This ongoing refinement enhances user engagement and contributes to heightened satisfaction.
Dynamic Content Recommendations
At the forefront of machine learning’s transformative influence on personalisation is the integration of dynamic content recommendation systems. These systems represent a visible and impactful manifestation of machine learning algorithms, meticulously analysing user preferences and behaviours to offer tailored suggestions for relevant content, products, or services. This dynamic adaptability is evident across diverse online platforms, whether streaming services proposing movies or e-commerce websites presenting product recommendations
The essence of these recommendation systems lies in their continuous learning capability. Embedded within these systems, machine learning algorithms absorb insights from user interactions, refining their understanding of individual tastes over time. This iterative process ensures the recommendations remain relevant and intricately aligned with each user’s preferences. Consequently, users are presented with a personalised selection that resonates authentically with their interests, fostering a heightened sense of satisfaction.
Beyond user satisfaction, the impact extends to user engagement and conversion rates. These recommendation systems encourage prolonged user interaction and conversion by consistently delivering content or products aligned with user interests. This symbiotic relationship between machine learning, dynamic content recommendations, and user satisfaction underscores the transformative potential of personalised online experiences, where users seamlessly discover content that captures their attention and aligns with their unique preferences.
Tailoring User Interfaces with ML
Within the intricate landscape of web development, user interfaces (UI) are pivotal architects of the overall digital experience. Machine learning (ML) emerges as a transformative force, significantly shaping UI personalisation by orchestrating dynamic adaptations in layouts, features, and visual elements based on nuanced user preferences. This collaborative interplay between ML algorithms and UI components marks a departure from static design, ushering in an era of personalised interfaces that evolve in response to user behaviour.
ML’s contribution to UI lies in its ability to discern patterns from user interactions. By scrutinising how users engage with various UI components, ML algorithms gain insights into individual preferences, enabling them to optimise the layout for heightened user engagement. For instance, consider an e-commerce platform powered by machine learning that intuitively reorganises product displays based on a user’s historical browsing and purchase activities. This responsive approach tailors the user interface to align seamlessly with the user’s preferences, crafting a personal and intuitive shopping experience.
This adaptive UI paradigm, fostered by machine learning, transcends the conventional static design principles. Instead, it embraces a fluid and responsive framework, ensuring users encounter interfaces finely tuned to their preferences. In essence, the synergy between machine learning and UI personalisation redefines the contours of user interaction, making every click, scroll, and selection a seamlessly personalised journey within the digital realm.
Personalised E-Commerce Experiences
Within the expansive domain of e-commerce, the advent of machine learning has unfurled a new era of personalised experiences. Departing from the conventional one-size-fits-all approach to online shopping, machine learning (ML) algorithms have emerged as transformative agents, reshaping the fabric of e-commerce interactions. Analysing user behaviour, purchase history, and individual preferences, these algorithms empower e-commerce platforms to curate user journeys, introducing tailored product recommendations, customised discounts, and even individualised shopping interfaces.
80% of customers are more likely to purchase when brands offer personalised experiences. On the other hand, 66% of customers say that encountering content that isn’t personalised would stop them from purchasing. The transformative power of ML in e-commerce lies in its capacity to delve into the intricacies of user data. By scrutinising and deciphering patterns from user interactions, ML algorithms discern preferences, allowing e-commerce platforms to transcend generic offerings. For instance, consider a scenario where an online store dynamically adjusts its product recommendations based on a user’s browsing history and previous purchases, offering a selection finely tuned to individual tastes.
Moreover, the impact extends beyond personalised suggestions. Machine learning enables e-commerce platforms to craft individualised shopping interfaces, ensuring users encounter layouts and features aligned with their preferences. This adaptive approach enhances the overall user experience, catalyses increased conversion rates and heightened customer loyalty. Users are more likely to engage with and convert on a platform that understands their preferences and caters to them in a personalised and intuitive manner. Machine learning and e-commerce personalisation pave the way for a retail landscape where every interaction is a uniquely tailored experience.
Behavioral Analysis for Personalisation
Behavioural analysis emerges as a cornerstone in personalised web development, and machine learning is a proficient conductor in this symphony. With an innate ability to track and interpret user interactions, machine learning (ML) algorithms are pivotal in unravelling behavioural patterns, predicting future actions, and dynamically adapting websites to cater to individual preferences.
ML’s behavioural analysis prowess is its ability to discern subtle nuances from user interactions. By meticulously observing how users engage with a website, ML algorithms gain insights into their preferences, paving the way for a predictive model that anticipates future behaviors. For instance, envision an online news portal that tailors its content feed based on the genres and topics a user frequently explores, sculpting a curated news experience tailored to individual interests.
Furthermore, behavioural analysis extends beyond predicting user preferences; it becomes a proactive force in enhancing the overall user journey. By foreseeing potential user actions, ML algorithms enable websites to present relevant information, products, or services preemptively. This forward-looking approach transforms the user experience from reactive to anticipatory, ensuring that users are met with precisely what they need before explicitly seeking it. Whether suggesting related articles, products, or services, integrating behavioural analysis through machine learning elevates the online journey, creating an environment where every user interaction is met with personalised and contextually relevant content.
Conclusion
In summary, the integration of machine learning is a transformative force in the vast landscape of web development, playing a pivotal role in reshaping the personalisation paradigm. Machine learning algorithms have emerged as instrumental tools for developers, from the intricacies of user profiling and the dynamism of dynamic content recommendations to the nuanced tailoring of user interfaces and the revolutionary shifts in e-commerce experiences. These algorithms empower websites to transcend the static confines of traditional development, creating dynamic digital spaces that adapt and evolve in harmony with individual users.
The collective impact is evident in creating online environments that transcend generic interactions, offering a more engaging, satisfying, and intuitive experience. Machine learning’s ability to discern patterns, predict behaviours, and refine user profiles ensures that the digital landscape resonates authentically with users personally. As technology continues its relentless advancement, the symbiotic relationship between machine learning and web development is not only a current reality but also a harbinger of future possibilities.
FAQs
Q. How does machine learning improve content recommendations?
A. To understand patterns, machine learning algorithms analyse user behaviour, preferences, and interactions. It enables dynamic content recommendations by predicting what users will likely be interested in based on past actions. The continuous learning process ensures that recommendations stay relevant and personalised over time.
Q. Can machine learning be applied to any website?
A. Machine learning can be applied to various websites, from e-commerce platforms and content streaming services to news portals and social media. The adaptability of machine learning algorithms allows developers to tailor personalisation strategies based on different websites’ specific needs and goals.
Q. How does machine learning contribute to e-commerce personalisation?
A. In e-commerce, machine learning enhances personalisation by analysing user behaviour, purchase history, and preferences. This data provides personalised product recommendations, customised discounts, and individualised shopping interfaces, creating a more engaging and satisfying user shopping experience.